Enter prompt to debloat:
Example Prompts
What is this?
Prompt Debloat helps you quickly identify which parts of your prompt matter most—and which you can safely remove—so you can streamline your prompts without sacrificing output quality. Optimize your prompt engineering by visualizing token importance. Get better results from your LLM with fewer tokens, saving costs and reducing environmental impact.
Inspired by @sama's humorous but insightful (and probably accurate) comment about the hidden cost of 'please' and 'thank you' tokens, we built Prompt Debloat to spotlight unnecessary fluff. While extra tokens seem harmless when chatting casually with ChatGPT, they can add significant costs and inefficiencies when repeated millions of times in production. Prompt Debloat reveals exactly which tokens your LLM actually cares about—helping you craft leaner, more efficient prompts.
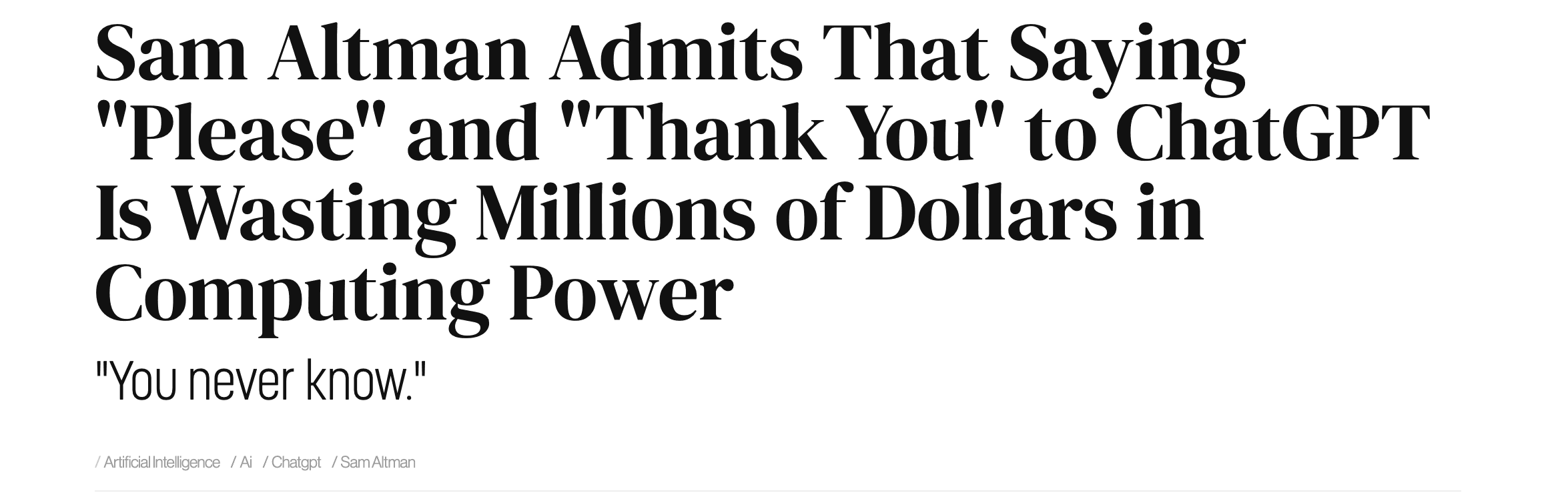
How does it work?
Prompt Debloat leverages a technique called token ablation, also known as input perturbation, to measure the importance of each token in your prompt. At a high level, the method works by iteratively removing tokens from your original prompt and observing how these changes affect the model's output.
By systematically omitting each token, Prompt Debloat identifies which tokens significantly impact the LLM's response and which ones have minimal influence. Tokens that do not substantially alter the output when removed can be considered 'bloat' and safely eliminated, resulting in more concise and cost-effective prompts.
What is a Token?
A token is a piece of text that language models use to process and generate text. Tokens can be individual words, parts of words, punctuation marks, or special characters. Language models like GPT-4 interpret your input by breaking it down into these discrete tokens, which are then analyzed and processed internally to produce an output.
What are Log Probs?
Log probabilities (logprobs) measure how likely the model believes a specific token or sequence of tokens is to occur next, given your input prompt. A high logprob indicates that the model strongly expects that token, whereas a low logprob means the token is less likely or more surprising to the model. By monitoring changes in logprobs when tokens are removed, Prompt Debloat assesses the significance of each token.
Limitations of Token Ablation
- Context Dependency: Token importance can vary depending on the context, so a token deemed unnecessary in one scenario might be crucial in another.
- Combinatorial Explosion: Testing all possible combinations of tokens rapidly becomes infeasible as prompt length increases, limiting the thoroughness of ablation studies.
- Subtlety of Impact: Some tokens might subtly affect the model's response in nuanced ways not fully captured by logprob differences alone.
Alternative Approaches for Prompt Debloating
- Gradient-based Methods: Using model gradients to identify token importance can offer more precise insights, though it requires deeper model access.
- Feature Attribution Methods: Techniques such as integrated gradients or SHAP values provide a more nuanced understanding of token importance by attributing model outputs directly to input tokens.
- Prompt Optimization Models: Specialized smaller models or supervised methods trained explicitly for prompt simplification can automate and potentially improve upon token ablation results.